Eyes Wide Shut: Exploring The Visual Shortcomings Of Multimodal LLMs
In the rapidly advancing world of artificial intelligence, multimodal Large Language Models (LLMs) have emerged as powerful tools capable of processing and generating both textual and visual content. However, despite their impressive capabilities, these models are not without limitations. One of the most critical areas where multimodal LLMs face significant challenges is in their visual processing capabilities. Understanding these shortcomings is crucial for developers, researchers, and users alike, as it highlights the need for further advancements in AI technology.
The integration of visual data into language models opens up a wide array of possibilities, from generating realistic images to interpreting complex visual information. However, the limitations in visual processing can hinder the effectiveness of these models, impacting their ability to provide accurate and meaningful outputs. As we delve deeper into this topic, we will explore the specific challenges faced by multimodal LLMs in handling visual data and the potential implications for various industries.
This article aims to provide a comprehensive analysis of the visual shortcomings of multimodal LLMs, offering insights into the current state of AI technology and the areas that require further development. By understanding these limitations, we can better appreciate the progress that has been made and the work that still lies ahead in creating more robust and versatile AI models.
Read also:Johnny Manziel Shower Exploring The Phenomenon And The Man Behind It
What Are Multimodal LLMs?
Multimodal LLMs are advanced AI models designed to process and generate content across multiple modalities, including text, images, audio, and video. Unlike traditional language models that focus solely on text, these models can integrate information from various sources to produce richer and more comprehensive outputs.
These models are built using deep learning techniques and are trained on vast datasets containing both textual and visual information. This allows them to understand the relationships between different types of data and generate outputs that combine elements from multiple modalities.
Key Features:
- Text and Image Integration
- Cross-Modal Understanding
- Enhanced Contextual Awareness
The Rise of Multimodal AI
The development of multimodal LLMs has been driven by the increasing demand for AI systems that can handle diverse data types. As industries such as healthcare, education, and entertainment seek to leverage AI for more advanced applications, the need for models capable of processing multiple modalities has become more pressing.
Visual Processing in Multimodal LLMs
Visual processing is one of the core functionalities of multimodal LLMs. These models are designed to interpret and generate visual content, enabling them to perform tasks such as image captioning, visual question answering, and image synthesis.
However, despite their capabilities, multimodal LLMs often struggle with certain aspects of visual processing. These challenges can impact the accuracy and reliability of their outputs, limiting their effectiveness in real-world applications.
Read also:Dakota Tyler Today The Journey Achievements And Impact
Challenges in Visual Processing
Some of the key challenges faced by multimodal LLMs in visual processing include:
- Interpreting Complex Visual Data
- Handling Ambiguities in Images
- Generating High-Quality Visual Outputs
Limitations of Multimodal LLMs in Visual Tasks
While multimodal LLMs have made significant strides in visual processing, they still face several limitations that hinder their performance. These limitations are primarily related to their ability to accurately interpret and generate visual content.
One of the main issues is the models' difficulty in understanding the context and nuances of visual data. This can lead to errors in image interpretation and generation, affecting the overall quality of their outputs.
Key Limitations:
- Lack of Contextual Understanding
- Difficulty in Handling Fine-Grained Details
- Challenges in Recognizing Rare or Uncommon Objects
Impact on Real-World Applications
The visual shortcomings of multimodal LLMs have significant implications for various industries that rely on AI for visual processing tasks. From healthcare to autonomous vehicles, the limitations of these models can impact the effectiveness and safety of AI-driven solutions.
In healthcare, for example, the inability of multimodal LLMs to accurately interpret medical images can lead to misdiagnoses and delayed treatment. Similarly, in the automotive industry, the limitations in visual processing can affect the performance of self-driving cars, potentially compromising passenger safety.
Industries Affected:
- Healthcare
- Automotive
- Entertainment
Current Research and Development Efforts
Researchers and developers are actively working to address the visual shortcomings of multimodal LLMs. Various approaches are being explored to improve the models' ability to process and generate visual content accurately and reliably.
Some of the key areas of focus include enhancing the models' contextual understanding, improving their ability to handle fine-grained details, and developing more robust training datasets.
Notable Advances:
- Improved Contextual Understanding
- Enhanced Fine-Grained Detail Recognition
- Development of More Robust Training Datasets
Future Directions for Multimodal LLMs
As research and development efforts continue, the future of multimodal LLMs looks promising. Advances in AI technology and the integration of new methodologies are expected to lead to significant improvements in the models' visual processing capabilities.
Some of the potential future developments include the incorporation of more advanced neural architectures, the use of larger and more diverse training datasets, and the development of new algorithms specifically designed for visual processing tasks.
Potential Developments:
- Advanced Neural Architectures
- Larger and More Diverse Training Datasets
- New Algorithms for Visual Processing
Conclusion
In conclusion, while multimodal LLMs have made remarkable progress in integrating textual and visual data, they still face significant challenges in visual processing. Understanding these limitations is crucial for advancing AI technology and developing more robust and versatile models.
We invite you to share your thoughts and insights on this topic in the comments section below. Additionally, feel free to explore other articles on our site for more in-depth analyses of AI technology and its applications.
Table of Contents
- What Are Multimodal LLMs?
- Visual Processing in Multimodal LLMs
- Limitations of Multimodal LLMs in Visual Tasks
- Impact on Real-World Applications
- Current Research and Development Efforts
- Future Directions for Multimodal LLMs
- Conclusion
Subheadings
- The Rise of Multimodal AI
- Challenges in Visual Processing
- Industries Affected
- Notable Advances
- Potential Developments
Unveiling The Jonathan Scott Tragedy 2023: A Story That Shook The World
Breaking The Quiet Full: Unveiling The Power Of Speaking Up
Emma Langevin Feet: A Comprehensive Exploration
Eyes Wide Shut Exploring The Visual of Multimodal LLMs
Eyes Wide Shut? Exploring the Visual of Multimodal LLMs
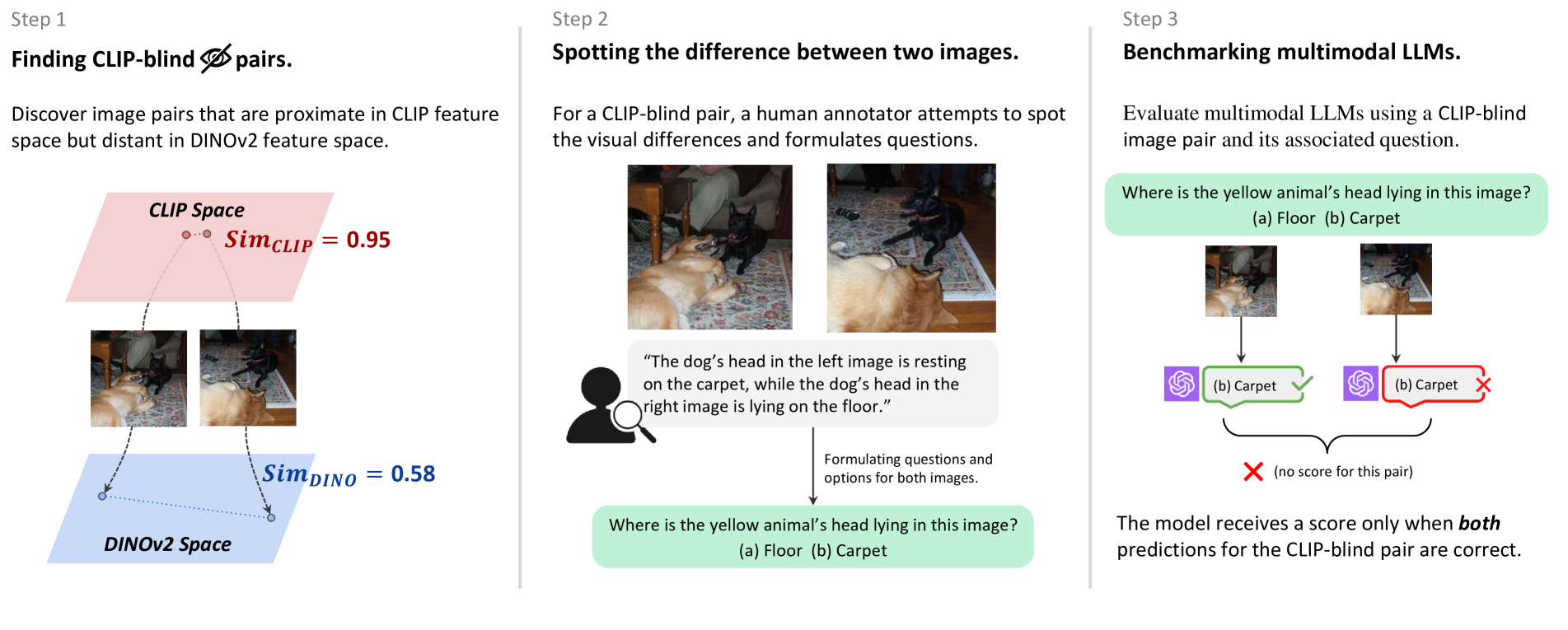
Eyes Wide Shut? Exploring the Visual of Multimodal LLMs